Module 2: What is AI and Why It Matters in Business
AI-Powered Business & Management Mastery
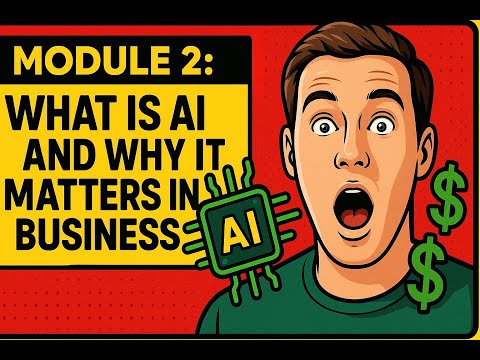
AI-Powered Business & Management Mastery
Imagine you’re the CEO of a global tech firm. You’re on a video call with your team from five continents, facing a complex decision about launching a new product. You need answers – fast. Suddenly, your AI dashboard updates in real-time: market sentiment, competitor moves, customer behavior trends – all synthesized into a simple recommendation. You make a confident decision. In that moment, it’s not just you leading – it’s the power of Artificial Intelligence transforming the future of business and management.
Welcome to AI-powered decision-making, machine learning, and deep learning. If you’re a manager, entrepreneur, student, or executive – this episode is tailored for you.
The Power of AI in the Startup Trenches Let me tell you about a small Indian fintech startup, FinSense. In 2020, they were struggling to break into a crowded market. But their secret weapon? A machine learning engine trained on micro-behavioral data of underserved rural users. Within six months, FinSense achieved a 73% increase in loan repayment rates and attracted venture capital. Their AI didn’t just analyze data – it created a competitive edge.
Fortune 500 Decisions on Autopilot Now contrast that with a Fortune 500 consumer goods company in Germany. Their global supply chain faced disruptions during the pandemic. But thanks to deep learning models predicting raw material shortages weeks in advance, they avoided millions in losses. In their boardroom today, AI doesn’t sit outside – it has a seat at the table.
Understanding AI, Machine Learning & Deep Learning Let’s decode the hierarchy:
Artificial Intelligence (AI) is the broad concept – it simulates human intelligence. Think of it as the umbrella term.
Machine Learning (ML) is a subset of AI. It enables systems to learn from data and improve without being explicitly programmed.
Deep Learning (DL) is a subset of ML that uses neural networks – mimicking the human brain – to solve highly complex problems.
In short, it’s a nested hierarchy: AI > ML > DL.
Understanding AI, Machine Learning & Deep Learning – A Simple Breakdown with Examples
Let’s Decode the Hierarchy:
At its core, these technologies form a nested hierarchy: Artificial Intelligence (AI) > Machine Learning (ML) > Deep Learning (DL)
Artificial Intelligence (AI) – The Umbrella Concept
AI refers to any technique that enables machines to mimic human intelligence — such as reasoning, learning, problem-solving, perception, or even understanding language. AI can be thought of as the broad concept under which both machine learning and deep learning fall.
Example:
Imagine a virtual assistant that understands spoken language, schedules your tasks, answers questions, and reminds you of appointments. It simulates human-like behavior by processing your voice, understanding your intent, and responding appropriately. This is a very basic example of AI.
Key Traits of AI:
Decision-making
Natural language understanding
Visual recognition
Basic automation
Machine Learning (ML) – Learning from Data
Machine Learning is a subset of AI that allows systems to improve through experience (data), rather than being explicitly programmed for every task. A machine learning model “learns” patterns from data and uses these patterns to make predictions or decisions without direct human input. Essentially, it’s like teaching machines to improve based on experience.
Example:
A system that analyzes thousands of past weather reports and learns to predict the weather for tomorrow — based on trends like temperature, wind patterns, and humidity levels — is using machine learning. It identifies patterns in past data and uses those patterns to predict future outcomes.
Common ML Use Cases:
Email filtering to detect spam
Predicting stock market trends based on historical data
Fraud detection by analyzing spending patterns
Deep Learning (DL) – Neural Networks Inspired by the Human Brain
Deep Learning is a subset of Machine Learning that uses artificial neural networks, designed to mimic the way the human brain processes information. It’s particularly useful for solving highly complex problems, especially those involving unstructured data like images, audio, or text. Neural networks consist of layers of nodes (or “neurons”), and as data passes through each layer, the system refines its understanding, much like the way the human brain does.
Example:
Imagine a system trained on thousands of medical images, such as X-rays or MRIs. It can detect subtle patterns in the images, identifying early signs of disease that might be difficult for human doctors to spot. This type of system uses deep learning to analyze data, which makes it especially powerful for highly complex tasks.
Common DL Use Cases:
Facial recognition in images
Translating languages in real-time
Speech-to-text transcription
Autonomous vehicles analyzing the surrounding environment using camera feeds
A Simple Analogy:
AI is like teaching a robot to act smart — it learns to solve problems or take actions based on predefined instructions or logic.
ML is giving the robot access to data so it can learn from experience. Over time, the robot gets better at performing tasks based on patterns in the data it has processed.
DL is giving the robot a brain to solve much more complex problems. It processes information through multiple layers of neural networks, enabling it to understand complicated patterns, like recognizing faces or understanding speech.
Summary: Why This Matters
Understanding the hierarchy of AI, ML, and DL helps clarify how these technologies build upon each other.
AI provides the broad framework for machines to simulate human intelligence.
ML allows machines to learn from data and improve their performance over time.
DL takes this a step further by enabling machines to process complex data through neural networks, mimicking the way humans think.
Together, they represent the evolution of how machines are getting smarter and capable of performing increasingly sophisticated tasks that were once thought to be uniquely human.
Examples for Clarity:
AI: Chatbots answering customer service queries.
ML: A system predicting loan defaults based on user behavior.
DL: Self-driving cars learning from millions of road scenarios.
AI’s Impact on Business Decision-Making
Speed & Scale: AI enables rapid decisions based on terabytes of data across markets.
Bias Reduction: Algorithms detect and correct patterns without emotional interference.
Predictive Intelligence: Real-time insights support strategic decisions.
Enhanced Planning: AI assists in scenario modeling and long-term forecasting.
Customer Experience: From dynamic pricing to personalized journeys, AI boosts satisfaction and loyalty.
Got it! Here's a refined version of AI’s Impact on Business Decision-Making, without referencing specific brands or companies, but still rich with practical and relatable examples:
AI’s Impact on Business Decision-Making – Explained with Practical Examples
Speed & Scale: Instant Decisions from Massive Data
AI can analyze massive amounts of data — market trends, customer behavior, and internal performance metrics — in seconds, enabling decisions that would take humans days or weeks.
Example:
A retail business processes data from thousands of daily transactions, customer feedback, and competitor pricing. AI helps the team adjust inventory and pricing strategies instantly based on real-time demand spikes.
Bias Reduction: Emotion-Free, Data-Driven Judgments
Human decision-making often suffers from unconscious bias or emotional influence. AI systems use consistent logic, leading to more fair and objective outcomes.
Example:
An HR department uses AI to evaluate candidates based on skills, experience, and performance in simulated tasks. The process avoids favoritism or bias from names, accents, or appearances — promoting a more diverse and qualified workforce.
Predictive Intelligence: Anticipating What Comes Next
AI excels at recognizing patterns and forecasting future outcomes, helping businesses anticipate changes and act proactively.
Example:
A logistics team uses AI to predict delivery delays by analyzing traffic, weather, and historical data. This allows them to reroute shipments and inform customers in advance — improving service and reducing costs.
Another Use Case:
A subscription service uses AI to flag users who may cancel soon. This helps the marketing team intervene with personalized offers to retain those customers.
Enhanced Planning: Smarter Strategy with Scenario Modeling
AI helps simulate various future scenarios — like changes in customer demand, economic shifts, or supply chain disruptions — allowing leaders to plan more accurately.
Example:
A manufacturing company runs AI models to test what would happen if raw material prices rise or if a supplier shuts down. The leadership team uses these insights to create backup plans and protect profit margins.
Customer Experience: Personalization & Loyalty Building
AI enables businesses to offer customized experiences based on individual preferences, behavior, and needs — leading to deeper engagement and long-term loyalty.
Example:
An online store uses AI to recommend products based on a customer’s browsing habits, purchase history, and seasonal trends. It also adjusts pricing dynamically to match market demand — creating a personalized and efficient shopping experience.
Support Use Case:
A chatbot powered by AI handles routine customer queries instantly, freeing up human agents to manage complex issues. This improves customer satisfaction while reducing operational load.
Summary: Why AI is a Game-Changer for Decision-Making
Faster insights lead to faster actions
Reduced bias leads to fairer outcomes
Predictions lead to preparedness
Simulations lead to stronger strategies
Personalization leads to happy customers
Case Study 1 – XYZ Personalized Retail at Scale Amazon’s recommendation engine, powered by deep learning, contributes to 35% of its revenue. It analyzes browsing behavior, purchase history, and even mouse movement to suggest products. This is not just automation – it’s the monetization of predictive analytics.
Case Study 2 – XYZ: Revolutionizing Healthcare The XYZ uses AI in diagnostic imaging, helping radiologists identify cancer with greater precision. Their partnership with Google Health has enabled AI-driven diagnostics that improve accuracy while reducing physician burnout. That’s the difference AI makes – lives saved, efficiency increased.
Top 10 FAQs with Expert Answers
What’s the key difference between AI, ML, and DL? AI is the overarching field; ML is learning from data; DL uses neural networks for complex tasks.
Can AI replace managers? No – but it augments them. AI handles data; humans bring judgment, ethics, and creativity.
How can startups use AI affordably? Cloud-based AI APIs like Google Vertex, Azure AI, and Amazon SageMaker offer scalable, cost-effective solutions.
Is AI only useful for tech firms? Not at all. AI is transforming retail, agriculture, education, finance, logistics – virtually every sector.
How can I learn to integrate AI into my business? Start with platforms like Coursera, Udacity, or LinkedIn Learning. Partner with AI consultants or pilot low-risk projects.
Is data privacy a concern with AI? Absolutely. Implementing AI ethically means ensuring GDPR/CCPA compliance, anonymization, and secure data handling.
What skills do managers need in an AI-first world? Data literacy, strategic thinking, change management, and ethical leadership.
What are the top tools for AI in business? Popular tools include TensorFlow, IBM Watson, Salesforce Einstein, and DataRobot.
What’s the ROI on AI investments? McKinsey reports that AI adopters see up to 20% increase in EBITDA across sectors.
Can AI make real-time decisions? Yes – in areas like fraud detection, inventory management, and customer service automation.
Top 5 Actionable Tips
Invest in data infrastructure. Quality data fuels effective AI systems.
Start small. Run pilot programs before full-scale AI adoption.
Upskill your teams. Encourage AI literacy and continuous learning.
Partner smartly. Collaborate with AI startups, consultancies, or platforms.
Lead ethically. Align AI use with company values and compliance norms.
Summary Today, we explored the layered world of AI, ML, and DL. We uncovered how these technologies are transforming decision-making, saw real-world applications from Amazon to XYZ, and offered practical advice to bring AI into your business world.
AI is not a far-off concept. It’s here, now, embedded in everything from our product launches to our HR policies. And for those who harness it wisely, the rewards are monumental.
Disclaimer: This podcast is for informational purposes only. While every effort has been made to ensure accuracy, listeners are encouraged to consult experts before making strategic business or financial decisions based on the content.