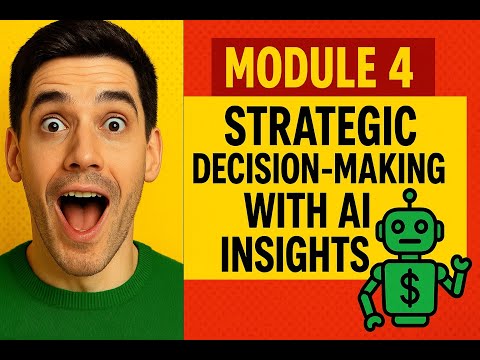
Module 4 Strategic Decision-Making with AI Insights AI-Powered Business & Management Mastery – A Complete Course
#AIForBusiness, #PredictiveAnalytics, #SmartDecisionMaking, #BusinessSuccess, #LeadershipTools, #GlobalStrategy,#EntrepreneurMindset, #DigitalTransformation, #InnovationInAction, #AITrends
In boardrooms from New York to Nairobi, from Mumbai to Munich, one question echoes louder than ever: "Are we making the right decisions fast enough?" In a world where markets shift in milliseconds, decisions based on gut instinct alone no longer cut it. Today, data is the new gold—and Artificial Intelligence is your most powerful mining tool.
Welcome to Module 4 of our global leadership podcast series, where we unravel how AI-driven strategic decision-making is transforming the way companies win—or lose—in today’s hyper-competitive landscape.
The Startup That Saw the Storm Before It Hit
Imagine a small e-commerce startup in Southeast Asia. They were competing with giants, struggling with cash flow, and trying to scale logistics. By leveraging AI to analyze customer behavior and market trends, they predicted a sharp rise in demand for hygiene products two weeks before the pandemic was officially declared. They restructured their inventory strategy overnight—and tripled their revenue in 30 days. They didn’t guess. They knew—because AI told them first.
The Hotel Chain That Avoided a Pricing Disaster
Now picture a global hotel chain in Europe. Traditionally, they set seasonal prices based on past bookings. But one year, something felt “off.” Their AI systems flagged unexpected booking patterns from travelers in specific countries—signals of political unrest and travel restrictions. The result? Dynamic pricing strategies were adjusted in real-time, avoiding millions in potential losses and reputational damage. Without AI, this would’ve gone unnoticed until it was too late.
Understanding Strategic Decision-Making with AI
Let’s break it down into three powerful applications of AI for business planning:
Predictive Analytics
At the core of strategic AI lies predictive analytics—the art of using historical data to foresee future events.
Forecast Trends: AI sifts through years of data, identifying hidden patterns humans might miss.
Opportunity Spotting: It surfaces new customer segments, demand shifts, and product gaps before competitors even notice.
Threat Detection: Early warnings for potential supply chain issues, policy changes, or customer churn patterns.
Module Example: A multinational beverage company used AI to predict seasonal flavor trends in emerging markets. By aligning production accordingly, they reduced waste and increased profitability.
Harnessing the Future: AI-Powered Predictive Analytics for Strategic Business Decisions
Imagine knowing what your customers will want before they even do. Picture a world where your inventory, pricing, and even marketing campaigns adjust themselves in real-time, anticipating changes before they occur. This isn’t science fiction—it’s the strategic power of AI-driven Predictive Analytics, and it’s reshaping the global business landscape at an unprecedented pace.
A mid-sized logistics company in Mumbai was constantly facing delayed deliveries and fluctuating fuel costs. Instead of reacting to disruptions, they implemented an AI-powered predictive analytics system that analyzed five years of traffic, weather, and seasonal demand data. The result? A 22% reduction in delivery time and 13% cost savings in operations within just six months. Forecasting empowered them to act, not just react.
A European fashion retailer used predictive analytics to identify that their customers were likely to shift from physical stores to online purchases during an upcoming winter season, driven by a combination of health concerns and historical weather patterns. They ramped up online inventory and optimized digital marketing in advance—leading to a 34% spike in online sales and reduced warehouse bottlenecks.
What is Predictive Analytics with AI?
Predictive analytics uses historical data, machine learning models, and statistical algorithms to predict future outcomes. When AI is infused into the process, it scales the analysis across massive datasets and continually learns to improve forecast accuracy.
How It Works – The Process Simplified:
Data Collection:
AI gathers structured and unstructured data from CRMs, ERPs, social media, customer reviews, IoT sensors, and more.
Data Cleaning & Preparation:
The system removes redundancies, fills in missing values, and normalizes data for modeling.
Feature Engineering:
AI identifies key factors—like customer age, product type, location—that most impact desired outcomes.
Model Training & Validation:
AI builds predictive models using algorithms like Random Forests, Neural Networks, or XGBoost and tests for accuracy.
Prediction & Insight Generation:
It forecasts trends such as demand, churn, pricing fluctuations, or supply chain risks—and gives actionable recommendations.
Real-Time Learning:
As new data enters, the AI refines and retrains itself, ensuring decisions are always data-driven and context-aware.
Company ABC’s Demand Forecasting
Company ABC employs AI-powered predictive analytics to optimize its inventory management. By analyzing customer behavior, search trends, delivery times, and market seasonality, it predicts product demand in different geographies. This reduces excess inventory, cuts storage costs, and ensures faster delivery, reinforcing Company ABC’s customer-first strategy.
Lesson: Predictive analytics helps anticipate what customers want, before they place the order—giving you a strategic edge.
Company XYZs & Customer Retention
Company XYZs uses AI to analyze historical transaction data to predict customer churn. The model looks at subtle behavior changes—like reduced usage frequency or payment pattern anomalies—and proactively offers loyalty programs or interventions. This led to a lower churn rate and significantly higher customer lifetime value (CLTV).
Lesson: Predictive analytics can save millions by preventing problems before they arise, not just solving them afterward.
Common Applications Across Industries:
Retail: Forecast inventory needs, optimize pricing, reduce cart abandonment.
Healthcare: Predict disease outbreaks, manage patient risk, reduce hospital readmissions.
Finance: Detect fraud, assess credit risk, forecast loan defaults.
Manufacturing: Predict equipment failures, improve supply chain planning, reduce downtime.
Tourism & Hospitality: Forecast seasonal demand, personalize offers, optimize staffing.
10 Actionable Tips for Using AI in Predictive Analytics:
Start with Clean, High-Quality Data – Garbage in, garbage out. Quality data is the backbone.
Define Clear Business Objectives – Know what you’re predicting and why (e.g., churn, demand, risk).
Leverage Cross-Functional Teams – Data scientists, marketers, and domain experts must collaborate.
Use Open-Source Frameworks – Tools like Python, R, and TensorFlow are flexible and cost-effective.
Avoid Overfitting Models – Balance model accuracy with generalization to unseen data.
Regularly Update Your Models – AI learns over time—keep models current to stay accurate.
Visualize Insights Clearly – Dashboards and heatmaps help stakeholders act on predictions.
Incorporate External Data Sources – Weather, news, competitor pricing add context and precision.
Ensure Ethical Data Use – Respect privacy, comply with data protection laws like GDPR.
Pilot First, Scale Fast – Test on a small scale, measure ROI, then roll out enterprise-wide.
AI in SWOT & Risk Analysis
Traditional SWOT analysis relies heavily on subjective team insights. AI revolutionizes this by bringing real-time, data-backed clarity.
Internal Strengths/Weaknesses: Automates analysis of operational efficiency, employee sentiment, and financial performance.
External Opportunities/Threats: Tracks macroeconomic trends, social sentiment, regulatory changes—instantly.
Risk Management: Flags anomalies, suggests mitigation plans, and prioritizes responses based on impact.
Module Example: A logistics company layered AI over global shipping data and government advisories, allowing for real-time SWOT recalibration during port strikes—avoiding critical delivery delays.
AI in SWOT & Risk Analysis: The New Era of Intelligent Strategic Planning
When it comes to making strategic business decisions, SWOT analysis—understanding Strengths, Weaknesses, Opportunities, and Threats—has long been a foundational tool. But today, we're witnessing a transformation: AI is not just participating in this process; it’s elevating it to a real-time, data-powered strategic advantage.
Let’s explore how.
The Traditional vs. AI-Driven SWOT: What’s Changed?
Traditionally, SWOT analysis was manual, static, and subjective, often based on leadership’s opinions, market assumptions, or quarterly reports. The insights were often outdated by the time they were implemented.
Now, AI enables:
Real-time internal and external environment scanning
Automated pattern detection
Scenario forecasting and risk modeling
Data-backed SWOT factors for increased precision
Example: Imagine a retail chain evaluating expansion. Traditional SWOT might identify internal strength as “strong brand presence” and a threat as “increasing competition.” With AI, those insights become layered with real-time competitor pricing trends, customer sentiment analysis, and even location-based spending behavior—updated continuously.
How AI Powers Each Element of SWOT
Let’s break it down:
Strengths & Weaknesses (Internal Factors)
AI tools analyze internal performance metrics, customer feedback, employee productivity, inventory efficiency, and more.
Natural Language Processing (NLP) reads internal emails or feedback to identify pain points.
AI dashboards provide operational health scores and predictive KPIs.
Example: An enterprise SaaS firm discovered its customer support was a key strength based on AI-powered analysis of support ticket resolution times and sentiment scores from reviews.
Opportunities & Threats (External Factors)
AI continuously mines market data, competitor moves, regulatory changes, and even geopolitical events to flag opportunities and risks.
Web scraping bots pull competitor data.
Machine learning models forecast market shifts before they happen.
Example: A fintech startup used AI to identify emerging trends in contactless payments post-pandemic by analyzing millions of social media posts and financial transactions—flagging it as a growth opportunity months before competitors caught on.
A European Telecom Leader
Faced with declining market share, this company integrated AI into its strategic planning.
Internal data analysis identified strengths in customer service and weaknesses in outdated infrastructure.
External AI engines tracked competitor pricing and bundled offers across multiple platforms.
The result? A refined strategy that led to a 12% revenue increase within 9 months.
Global Insurance Provider
The firm implemented AI for dynamic risk modeling in 15 markets.
AI analyzed climate data, regional crime trends, and economic patterns to identify potential threat zones for new product launches.
SWOT updates were automated every week, not quarterly.
Outcome: Faster market entry, reduced fraud rates, and improved customer satisfaction.
Risk Analysis with AI: A Deeper Dive
Risk analysis often deals with what-ifs—scenarios with heavy consequences. AI helps here by:
Predictive analytics for risk identification
(e.g., supply chain disruptions based on satellite and news data)
Prescriptive modeling for mitigation strategies
(e.g., scenario simulation on alternative supplier routes)
Early warning systems for fraud, market crashes, or PR crises
Example: A logistics firm used AI to monitor global weather patterns, port congestion data, and geopolitical tensions to redirect shipping routes before disruptions occurred, saving millions in potential losses.
The Step-by-Step Process of Implementing AI in SWOT & Risk Analysis
Here’s how you can get started:
Step 1: Define your objectives
Are you planning market entry, cost-cutting, or new product development?
Step 2: Gather historical and real-time data
Use internal CRMs, ERP systems, social media, financial news, and competitor data.
Step 3: Integrate AI platforms for analysis
Feed data into AI models to categorize it into SWOT buckets.
Step 4: Set alerts and risk thresholds
AI should notify you when data signals a significant change.
Step 5: Review AI-generated insights with human oversight
AI brings the data. You bring the strategy.
Actionable Tips for Business Leaders and Teams
Use real-time dashboards for constant SWOT refresh cycles.
Blend structured and unstructured data—think financials and tweets.
Assign human experts to validate AI outputs regularly.
Create “What-if” risk models for best/worst-case forecasting.
Embed AI into your quarterly strategy meetings—not just your IT department.
Frequently Asked Questions (FAQs)
Q1: Can AI replace human strategic thinking in SWOT?
No. AI augments decision-making by removing guesswork, not replacing strategic intuition.
Q2: How often should AI update SWOT analysis?
Ideally, in real-time or weekly—depending on your industry’s volatility.
Q3: Do I need big data for this?
Not necessarily. Even mid-sized data sets can generate strong AI-driven insights when well-structured.
Q4: How can AI detect hidden risks?
AI scans thousands of data points—from regulatory changes to customer churn signals—to flag anomalies you might miss.
Q5: What industries benefit most?
Finance, insurance, retail, healthcare, logistics, and any data-rich industry.
Q6: What are the risks of using AI in SWOT?
Over-reliance, data bias, and lack of human context. Always combine AI with human judgment.
Q7: Is this expensive to implement?
Open-source tools and cloud-based AI solutions make it affordable for even startups.
Q8: Can startups use AI for SWOT effectively?
Yes. Startups can use lightweight tools to track funding trends, competitor growth, and customer reviews.
Q9: What skills do teams need?
Basic data literacy, critical thinking, and the ability to interpret AI insights strategically.
Q10: How can I measure ROI?
Look at time saved, decision accuracy, and impact on KPIs like customer retention or revenue growth.
Key Takeaways & Tips
Top 5 Actionable Tips:
Incorporate AI into every strategic planning cycle
Automate your SWOT updates using AI-powered dashboards
Prioritize high-impact threats and emerging opportunities
Train your teams to interpret AI-driven insights
Continuously validate your strategy against real-time data
AI doesn’t just assist in SWOT and risk analysis—it supercharges it. From detecting internal inefficiencies to identifying market threats in real-time, AI transforms static SWOT into a living, breathing strategic framework. The combination of data, automation, and human intuition makes this approach unbeatable in today’s volatile business climate.
Competitor Intelligence with AI
Competitive intelligence is no longer about hiring researchers to comb through quarterly reports.
AI Monitors Everything: From pricing and promotions to social sentiment and ad spending.
Sentiment Analysis: AI tools parse thousands of online reviews, social posts, and industry news to gauge customer perception.
Real-Time Alerts: Notifies you when competitors launch new products, change strategy, or receive negative PR.
Module Example: A fashion retail firm used AI to monitor influencer campaigns and real-time reviews of rival brands. They adapted their marketing tone and outperformed on brand sentiment within a month.
Segment Title: Competitor Intelligence with AI – Outthink, Outperform, Outsmart
Imagine being able to predict your competitors’ next moves, adjust your pricing in real-time, or detect subtle shifts in consumer sentiment before it explodes across markets. With AI-powered tools, that’s no longer science fiction—it’s today’s competitive advantage.
Why Competitor Intelligence with AI Matters
In the hyperconnected, data-saturated global market, businesses that react lose. Businesses that predict and preempt win. Traditional competitive analysis relied on quarterly reports and outdated market surveys. Today, AI lets you:
Continuously track competitor campaigns, pricing, product launches, and customer feedback.
Extract signals from unstructured data—social media, reviews, press releases, app updates.
Model strategic shifts based on historical behavior, competitor positioning, and digital footprint.
Now let’s break it down through real-world stories, practical use cases, and top global strategies.
How a Global Retailer Outsmarted its Rivals with AI
A global fashion retailer—let’s call them StyleEdge—was struggling with market saturation and price wars. Instead of slashing prices blindly, they used AI-driven competitor intelligence tools that scraped and analyzed thousands of data points from rival websites, ad campaigns, social media sentiment, and customer reviews.
Here’s what they discovered:
A competitor was promoting a massive clearance sale on a specific denim line.
Sentiment analysis showed frustration among buyers due to stockouts and slow delivery.
Action taken: StyleEdge launched a targeted Instagram ad campaign for their own premium denim with faster delivery options and an "In Stock Now" badge. Result? A 47% surge in conversions in 3 days, and they captured the audience before their competitors could replenish inventory.
SaaS Startup Gains Market Edge Through Sentiment & Feature Tracking
A rising SaaS firm in project management used AI to track feature updates, pricing changes, and support reviews of three top competitors.
They set up:
AI bots to monitor GitHub commits and changelogs.
Natural language processing (NLP) to analyze user complaints and feedback on G2, Reddit, and Quora.
Machine learning models to correlate negative reviews with feature removals.
They found:
One competitor had quietly removed Gantt chart support, sparking customer backlash.
Another hiked prices for enterprise users, causing churn.
Strategic move: The startup launched a “Feature Comeback” campaign, promoted robust Gantt charting, and offered migration discounts for enterprise clients. This boosted trials by 62% in 30 days and locked in new contracts.
The AI-Driven Competitor Intelligence Process
Here’s how top-performing companies structure their AI-powered competitor intelligence strategy:
Define Strategic Objectives
What do you want to track? Pricing, features, sentiment, ad spend, hiring patterns?
Data Collection with AI Agents
Use bots and scrapers to collect structured and unstructured data across web, social, and app ecosystems.
Pattern Recognition via Machine Learning
Train models to identify competitor behavior shifts, sentiment dips, pricing anomalies, and emerging trends.
Visualization & Insights
Dashboards highlight threats and opportunities in real-time (think of heatmaps, trend lines, sentiment clouds).
Predictive Modeling
AI forecasts what competitors might do next, using past behavior patterns, seasonality, and market signals.
Real-Time Strategic Adjustment
Act fast—launch campaigns, adjust pricing, innovate features, shift messaging.
5 Actionable Tips to Use AI for Competitor Intelligence
Automate Social Listening
Use NLP tools to track keywords, hashtags, and sentiment related to your competitors on platforms like X (formerly Twitter), LinkedIn, and Reddit.
Monitor Pricing & Promotions Daily
Set up bots to log daily prices, bundle changes, and limited-time offers—especially during seasonal periods.
Track Hiring Patterns
AI can scan job boards and LinkedIn posts to detect new hiring sprees. A surge in “machine learning engineer” roles? They’re likely building something new.
Leverage Competitor Review Mining
Use sentiment analysis on app stores, Trustpilot, G2, etc., to uncover pain points you can exploit with tailored offers or improvements.
Predict Campaign Timings
Train models on past email and ad launch timings—spot patterns like product releases every quarter or price drops every November.
Common FAQs About Competitor Intelligence with AI
Can small businesses use AI for competitor intelligence?
Absolutely. Open-source tools and freemium plans offer robust scraping, NLP, and analytics without heavy investment.
Is it legal to scrape competitor data?
Yes, as long as you collect publicly available data without violating terms of service. Always consult legal counsel.
How often should I monitor competitors?
For dynamic industries, real-time tracking is ideal. For slower ones, weekly or biweekly updates may suffice.
Can AI detect unspoken consumer needs?
Yes—AI picks up patterns in queries, feedback, and complaints that humans may overlook, revealing hidden opportunities.
What AI techniques are commonly used?
NLP for sentiment, ML for pattern prediction, OCR for visual content scraping, and deep learning for ad trend modeling.
How do I start with zero data science experience?
Use intuitive platforms with pre-built dashboards or partner with an AI consultancy.
What KPIs should I track?
Competitor market share, price volatility, sentiment shifts, share of voice, campaign timing, and user churn indicators.
Can AI help in long-term strategic planning?
Definitely. Predictive modeling forecasts competitor moves, product launches, and pricing evolution over time.
What’s the risk of over-reliance on AI?
AI is a tool, not a strategy. Combine insights with human judgment, domain expertise, and creative instincts.
Which industries benefit most from competitor intelligence?
SaaS, eCommerce, retail, finance, travel, and tech—basically, any sector where agility, customer sentiment, and innovation drive success.
Conclusion: Transform Strategy with Smart Competitor Intelligence
Let’s recap the essentials:
AI amplifies strategic foresight by turning raw market noise into refined competitor insights.
You’re no longer reacting—you’re anticipating.
Whether you’re a startup, a growth-stage company, or a multinational, AI-enabled market intelligence is no longer optional—it’s the difference between surviving and leading.
Top 5 Actionable Takeaways
Adopt real-time competitor tracking using AI.
Use sentiment analysis to uncover consumer pain points.
Leverage predictive modeling to foresee competitive moves.
Integrate intelligence directly into pricing, marketing, and product decisions.
Continuously refine your AI models as your market evolves.
A Global Financial Institution’s AI-Driven Strategy Module
One of the world’s top banking groups integrated AI into their risk management system. Predictive modeling helped flag client credit risks, while sentiment analysis from financial news helped forecast market shifts. The result? A 17% reduction in bad loans and faster portfolio decisions.
Leading E-Commerce Platform in India
This platform used AI to perform competitor analysis across 700 product categories. The system offered dynamic pricing recommendations based on seasonality, ad campaigns, and customer feedback. Revenue rose by 22% in the next quarter. Their edge wasn’t size—it was insight.
Top 10 FAQs – Answered
What is strategic decision-making with AI?
It’s the process of using AI-powered insights to make informed, forward-looking business decisions.
Can small businesses leverage AI too?
Absolutely. Cloud-based AI tools make it accessible for startups and SMBs with flexible, pay-as-you-go models.
Is AI better than human intuition?
AI complements human judgment by offering data-driven clarity—especially for complex or large-scale decisions.
How accurate is predictive analytics?
While no prediction is perfect, AI improves accuracy dramatically through continuous learning and real-time updates.
What industries benefit most?
Finance, retail, logistics, healthcare, and manufacturing—though almost every sector can benefit from smarter decisions.
How is AI used in SWOT analysis?
AI automates data collection and analysis, removing human bias and adding real-time external inputs like trends and risks.
What ethical concerns arise with AI decision-making?
Bias in algorithms, data privacy, and over-reliance on machines. Regular audits and transparent AI models are crucial.
What skills do I need to work with AI tools?
Basic data literacy, business strategy understanding, and platform-specific training—technical coding isn’t always required.
Can AI detect black swan events?
Not always—but it can detect anomalies and early signals that indicate possible disruptions.
How do I start implementing AI in my strategy?
Begin with a small pilot in one department. Define clear KPIs, use cloud-based AI services, and scale based on success.
Top 5 Actionable Tips
Start with clearly defined problems where data already exists
Invest in AI tools that offer integration, scalability, and transparency
Align AI models with business KPIs—not just technical outcomes
Combine human expertise with AI insights to avoid blind spots
Continuously review AI decisions to improve model performance
In Summary
Artificial Intelligence is no longer a futuristic buzzword. It’s your co-pilot in navigating complexity, uncertainty, and competition. From predictive analytics to automated SWOT evaluations and AI-powered competitor analysis, the businesses thriving today are those using AI not just to survive—but to strategize.
10 Frequently Asked Questions (FAQs) – Answered
What’s the difference between traditional and AI-driven analytics?
Traditional analytics is descriptive; AI adds the predictive layer and automation for forward-looking insights.
Is AI in predictive analytics affordable for startups?
Yes. Many cloud-based tools offer scalable, pay-as-you-go models ideal for smaller businesses.
What data do I need to start predictive analytics?
Sales, customer interaction, inventory, marketing campaign data, and even social media engagement.
Can predictive models work in non-digital businesses?
Absolutely. Even offline businesses collect useful data—like footfall, transactions, or seasonal sales trends.
How often should models be retrained?
It depends on data volatility—monthly to quarterly for most, real-time for high-frequency applications like finance.
Do I need in-house AI experts?
Not always. Many platforms offer no-code/low-code solutions, or you can outsource to analytics partners.
How do I measure success?
Track KPIs like forecast accuracy, revenue impact, operational savings, or customer satisfaction uplift.
Can predictive analytics reduce business risk?
Yes, especially in areas like credit scoring, fraud detection, inventory forecasting, and demand planning.
Is AI prediction 100% accurate?
No model is perfect. Think of it as guidance—not gospel—to make smarter, faster decisions.
What industries benefit most from predictive analytics?
Finance, retail, healthcare, logistics, insurance, SaaS, and manufacturing top the list.
Top 5 Takeaways & Actionable Tips
Use AI to shift from reactive to proactive strategy.
Start small—pilot with one business area (like churn or demand).
Invest in data quality—AI is only as smart as the data it learns from.
Align predictive analytics with strategic KPIs.
Focus on actionability—ensure the insights lead to measurable outcomes.
In this Module 4 we explored how AI-driven predictive analytics is transforming global businesses. We discussed how it forecasts trends, reduces risk, boosts customer loyalty, and optimizes resources. Through anecdotes, industry use-cases, and expert-backed insights, we’ve seen how AI turns uncertainty into opportunity—giving you a compass for navigating the future of business.
Disclaimer
This podcast is for informational purposes only. It does not constitute financial, legal, or investment advice. All examples are illustrative and may be hypothetical or adapted from real cases for educational clarity.