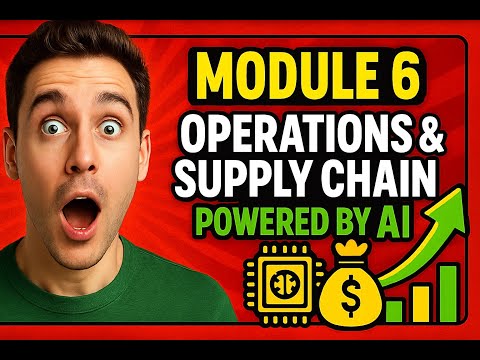
Module 6 Operations & Supply Chain Powered by AI
AI-Powered Business & Management Mastery
#AIinSupplyChain, #SmartLogistics, #InventoryManagement, #PredictiveAnalytics, #Automation, #FutureofWork, #Innovation, #Technology, #Business
We're diving into how Artificial Intelligence is reshaping the very heartbeat of global business logistics, boosting efficiency, cost savings, and customer satisfaction across industries.
Imagine a world where packages arrive at your doorstep almost before you even hit the "buy now" button. Where factories self-correct before errors occur. Where shelves never go empty, and supply chains are not only smart but predictive.
The Grocery Chain That Could Predict Demand
In 2023, a mid-sized grocery chain in Europe faced a familiar problem—frequent stockouts of fast-selling items and a surplus in others. Traditional methods, relying on historical data and manual forecasting, proved woefully inadequate in the face of rapidly changing consumer preferences and unpredictable external factors. By integrating an AI-powered demand forecasting system, the chain began to detect subtle shifts in consumer behavior, weather patterns, and even local events with remarkable precision. The AI system analyzed millions of data points, including social media trends, local event calendars, and real-time weather feeds, to predict demand at the individual store level. Within six months, their inventory turnover increased by 35%, and waste dropped by nearly 50%. This dramatic improvement not only boosted profitability but also enhanced the chain's reputation for reliability and customer service. It wasn’t magic—it was machine learning doing the heavy lifting.
Real-World Inspired: The Indian Apparel Startup That Automated to Scale
A rising Indian apparel startup, once overwhelmed by seasonal demand fluctuations and the complexities of a fragmented logistics landscape, turned to AI automation. The startup struggled with inaccurate demand predictions, leading to either stockouts and lost sales or excess inventory and increased storage costs. They also faced challenges in coordinating with multiple courier partners, resulting in delivery delays and inconsistent service quality. Predictive analytics suggested precise restocking timelines and optimal courier partners based on past performance, taking into account factors like delivery speed, cost-effectiveness, and reliability. By automating their supply chain with AI, the startup gained end-to-end visibility, optimized their inventory levels, and streamlined their logistics operations. They scaled 3X in under a year, all while reducing logistics costs by 22%. Their success story is now studied in top global MBA programs as a prime example of how AI can empower businesses in emerging markets to overcome logistical hurdles and achieve rapid growth.
AI is no longer a futuristic concept—it’s the backbone of modern business operations and supply chain management. From optimizing inventory levels to predicting demand and streamlining logistics, AI is transforming the way goods and services are produced, distributed, and consumed. Here’s how it’s making waves:
Inventory & Logistics Prediction
AI forecasts real-time demand using historical sales, customer behavior patterns, market trends, weather forecasts, and a wide range of economic indicators. This enables businesses to anticipate future needs with greater accuracy, moving away from reactive approaches to proactive planning.
Helps in reducing both overstock and stockouts, a major pain point for retailers and manufacturers alike. By minimizing these imbalances, companies can free up capital, reduce storage costs, and improve customer satisfaction.
Enhances route optimization through dynamic data—ensuring faster, greener, and cheaper deliveries. AI algorithms can analyze real-time traffic conditions, weather patterns, and delivery constraints to determine the most efficient routes, reducing fuel consumption and delivery times.
Breaking Down AI in Inventory & Logistics Prediction
Let’s start with a simple but powerful truth: In business, what you can’t predict, you can’t optimize. Inventory miscalculations cost companies billions of dollars annually—either by tying up capital in excess stock or losing customers due to stockouts. Traditional forecasting methods often fall short because they rely on historical data and fail to account for the complex interplay of factors that influence demand and supply. Now imagine having the ability to predict exactly what your customers want, when they want it, and how best to deliver it—all without manual guesswork. That’s where Artificial Intelligence steps in as a game-changer, offering unprecedented levels of accuracy and efficiency.
The Holiday Rush Crisis
Picture this: A mid-sized electronics retailer in the U.S. faced massive customer backlash during a major holiday season. The company, which had built its reputation on timely delivery and customer satisfaction, was caught off guard by an unexpected surge in demand for a particular product. Why? Their best-selling headphones ran out of stock two days into the sale. The inventory team relied on last year’s trends—but failed to account for viral social media buzz and influencer endorsements that suddenly spiked demand. This resulted in lost sales, frustrated customers, and damage to the retailer's brand image. After implementing AI-powered demand forecasting, the company not only met holiday demand but significantly increased revenue in that quarter alone. The AI system recognized social media patterns, regional buying behavior, and weather forecasts to predict demand spikes in real-time, allowing the retailer to proactively adjust its inventory levels and staffing.
From Chaos to Clarity in Rural India
Let’s shift our focus to a different context. In rural Maharashtra, a fast-growing agritech startup struggled with delivering fertilizers on time to farmers. The monsoon season made roads unpredictable, and farmers were often left waiting for critical supplies, impacting their crop yields and livelihoods. The startup faced the daunting task of coordinating deliveries across a vast and geographically diverse region with limited infrastructure and unreliable transportation. By integrating AI route prediction and demand mapping, delivery times dropped significantly, and customer satisfaction surged. The AI system analyzed a variety of data sources, including real-time satellite imagery of road conditions, hyper-local weather forecasts, and farmers' historical purchase patterns, to predict who would order what, and when—ensuring faster delivery and smarter inventory staging at micro-warehouses strategically located throughout the region.
Step-by-Step: How AI Powers Inventory & Logistics Prediction
So, how does this magic happen? Let's break it down into a step-by-step process:
Real-Time Demand Forecasting: AI models analyze massive datasets from past sales, customer behavior patterns, social media trends, economic indicators, and even granular weather data. These models can identify complex relationships and patterns that would be impossible for humans to discern, leading to more accurate predictions. For example, a beverage company uses AI to anticipate increased demand for cold drinks not just before a general heatwave, but also in specific neighborhoods experiencing localized spikes in temperature, allowing for highly targeted inventory adjustments.
Predictive Inventory Management: AI balances stock levels across the entire supply chain, from raw materials to finished goods, identifying what to reorder, when to reorder, and in what quantities. This goes beyond simple reorder points and considers factors like lead times, supplier reliability, and potential disruptions. Imagine an apparel retailer using AI to predict that a particular style of jeans will be not only generally popular in urban areas during back-to-school season but also that certain sizes and colors will be in higher demand in specific demographic segments.
Dynamic Route Optimization: AI-powered logistics platforms identify the fastest, safest, and most fuel-efficient delivery routes in real-time, taking into account a multitude of dynamic factors. This includes not only current traffic conditions and weather patterns but also unexpected events like accidents, road closures, and even local events that may cause delays. Delivery companies use AI to reroute drivers mid-day based on these factors, and also to optimize delivery sequences to minimize mileage and fuel consumption.
Inventory Heatmaps and Smart Warehousing: AI visualizes "hot zones" for inventory movement within warehouses and automates shelf restocking and order fulfillment processes. This involves using AI-powered robots and automated guided vehicles AGVs to optimize the placement of fast-moving items, predict optimal picking routes for warehouse workers, and even anticipate when shelves will need to be restocked, significantly reducing the time and effort required for warehouse operations.
But how can businesses leverage these powerful tools to transform their operations and gain a competitive edge?
Actionable Tips for Implementing AI in Inventory & Logistics Prediction
Here are some actionable tips for businesses looking to implement AI in their inventory and logistics operations:
Start with Data Integration: Unify your sales, warehouse management systems, customer relationship management CRM data, and third-party data sources. Clean, structured, and comprehensive data is the foundation of effective AI prediction.
Use Cloud-Based AI Tools: Explore the wide range of cloud-based AI platforms that offer scalable and cost-effective solutions for businesses of all sizes. These platforms provide pre-built AI models and tools that can be easily customized and integrated into existing systems.
Apply Predictive Analytics in Micro-Segments: Don't just forecast broadly—segment your customer base, product lines, and geographic regions to identify specific patterns and trends. This granular approach allows for more precise and targeted inventory management and logistics strategies.
Combine AI with IoT for Real-Time Feedback: Integrate AI systems with Internet of Things IoT devices, such as sensors and RFID tags, to gather real-time data on inventory levels, location, and condition. This enables continuous monitoring and dynamic adjustments to optimize operations.
Invest in Skills Before Systems: Prioritize training and upskilling your workforce in data literacy and AI fundamentals. Equip your employees with the knowledge and skills they need to effectively use and manage AI-powered systems, ensuring smooth adoption and maximizing ROI.
Global Impact: AI-Driven Logistics in Action
To truly understand the transformative impact of AI, let's look at some examples of how it's being used across the globe.
E-commerce Giant's Predictive Inventory
An e-commerce giant has revolutionized its logistics operations by using AI to predict what products customers are likely to buy—and shipping them to fulfillment centers and distribution hubs strategically located closer to those customers even before they click 'Buy Now.' This "anticipatory shipping" strategy minimizes shipping times, reduces return rates, and enhances the overall customer experience. AI also plays a crucial role in identifying hyper-local and region-specific buying trends, allowing the company to optimize its inventory at a granular level and tailor its product offerings to meet the unique demands of different markets.
Asian Retailer's Autonomous Logistics Ecosystem
An Asian retailer has built one of the world's most advanced AI-powered logistics ecosystems, integrating a wide range of cutting-edge technologies to automate its supply chain operations. Their system predicts demand with remarkable accuracy at the regional and even city level, schedules autonomous vehicles, including drones and robots, for last-mile delivery, and automates the entire restocking process. During major online sales events, this retailer uses AI to process the vast majority of orders with minimal human intervention, delivering products to customers across the country with remarkable speed and efficiency.
Now, let's tackle some of the most common questions businesses have about implementing AI in their inventory and logistics operations.
FAQs: Your Questions Answered
Here are ten frequently asked questions, with insightful and actionable answers:
Q: How does AI improve inventory forecasting accuracy compared to traditional methods? A: AI processes a much wider range of data sources, including real-time information and unstructured data, and uses advanced machine learning algorithms to identify complex patterns and relationships, leading to significantly higher forecasting accuracy—often exceeding 95% accuracy.
Q: Is AI cost-effective and accessible for small and medium-sized businesses, or is it only for large enterprises? A: Yes, AI is increasingly cost-effective and accessible for businesses of all sizes. The rise of cloud-based AI platforms and SaaS solutions has democratized access to AI tools, with many providers offering flexible pricing models and solutions tailored to the specific needs and budgets of SMEs.
Q: What is the typical ROI and payback period for implementing AI in logistics and supply chain management? A: The ROI for AI in logistics can vary widely depending on the specific implementation and the existing inefficiencies in a company's operations. However, studies have shown that businesses can achieve significant cost savings, ranging from 20% to 400%, and typically see a payback period of 6 to 18 months.
Q: How long does it typically take to implement AI in operations and supply chain management? A: The implementation timeline can vary depending on the complexity of the project and the scope of the AI solution. A basic rollout, such as implementing AI for demand forecasting in a specific product line, can take 2-6 months, while larger, integrated systems that involve multiple departments and processes may require up to a year or more.
Q: Will AI replace human jobs in supply chains, or will it augment human capabilities? A: AI is more likely to augment human capabilities rather than replace them entirely. While AI can automate routine and repetitive tasks, it also creates new opportunities for humans to focus on higher-level responsibilities that require creativity, critical thinking, and strategic decision-making. The roles of supply chain professionals will evolve, shifting from manual tasks to managing and optimizing AI systems.
Q: How is AI used in predictive maintenance, and what are the benefits? A: In predictive maintenance, sensors are used to collect data from equipment and machinery, and this data is then fed into machine learning models. These models can detect subtle anomalies and patterns that indicate potential failures, allowing businesses to schedule maintenance proactively, before breakdowns occur. This results in reduced downtime, lower repair costs, and extended equipment lifespan.
Q: Can AI help businesses mitigate the impact of global shipping delays and supply chain disruptions? A: Yes, AI can play a crucial role in mitigating the impact of disruptions. AI-powered systems can model and simulate the effects of various disruptions, such as port congestion, natural disasters, and geopolitical events, and identify alternate shipping routes, transportation modes, or suppliers dynamically. This enables businesses to respond to disruptions more quickly and effectively, minimizing their impact on operations.
Q: What are the key skills needed for supply chain professionals to effectively manage and work with AI systems? A: To effectively manage and work with AI systems, supply chain professionals need a combination of technical and business skills. Key skills include basic data literacy, an understanding of AI tools and techniques, proficiency in data analysis and interpretation, and the ability to align AI initiatives with overall business strategy.
Q: Are there any specific data privacy concerns that businesses should consider when implementing AI in supply chains, especially when dealing with customer or supplier data? A: Yes, data privacy is a critical consideration. Businesses must ensure that their AI implementations comply with relevant data protection regulations, such as GDPR and CCPA. This involves implementing robust data security measures, obtaining necessary consent for data collection and use, and ensuring transparency in how data is being used by AI systems.
Q: What is the best way for a business to get started with implementing AI in its operations and supply chain? A: The best approach is to begin with a specific, well-defined use case, such as demand forecasting for a particular product line or route optimization for a specific delivery area. This allows businesses to test the technology, measure the ROI, and gain valuable experience before scaling up to more complex and enterprise-wide AI implementations. Partnering with experienced vendors that offer AI integration solutions and support can also be beneficial.
To summarize, Artificial Intelligence is not just automating tasks; it's fundamentally reshaping the entire landscape of operations and supply chain management—making them more predictive, agile, on-demand, and efficient. From optimizing inventory levels and streamlining logistics to enhancing demand forecasting and enabling proactive risk management, AI is empowering businesses to make smarter decisions, operate more efficiently, and deliver greater value to their customers. Whether you're a startup founder disrupting an industry, a seasoned supply chain manager at a global corporation, or a student preparing for a career in this dynamic field, understanding the transformative power of AI is essential for success in the modern business world.
Key Takeaways: Actionable Insights for Leaders
Here are my top five takeaways for business leaders looking to leverage AI in their operations:
Leverage AI Now—Don’t Wait for Perfection: The technology is here, and the potential benefits are immense. Start exploring AI solutions and identify opportunities to implement them in your operations. Don't wait for the perfect solution or a future when the technology is more mature.
Think Modular and Incremental: You don’t need to automate everything at once. Begin with a specific use case or a pilot project to test the waters and demonstrate the value of AI. Then, gradually expand your AI initiatives to other areas of your operations.
Invest in Training and Upskilling Your Workforce: Your employees are your most valuable asset. Provide them with the training and resources they need to adapt to the changing landscape and work effectively alongside AI-powered systems. Foster a culture of continuous learning and experimentation.
Use Data as a Strategic Asset: Ensure you have a robust data management strategy in place. Collect clean, structured, and relevant data from across your organization and external sources. Invest in the tools and infrastructure needed to store, process, and analyze this data effectively.
Measure, Iterate, and Optimize: Continuously track key performance indicators KPIs to measure the impact of your AI initiatives. Use the insights you gain to refine your AI models, optimize your processes, and identify new opportunities for improvement.
Remember, the future of work isn't a distant horizon; it's unfolding before our very eyes, and it's being powered by the incredible potential of Artificial Intelligence. By embracing this technology and integrating it strategically into your operations, you can unlock unprecedented levels of efficiency, drive innovation, and gain a sustainable competitive advantage in the global marketplace. Stay ahead of the curve, embrace the change that's inevitable, and elevate your business to new heights of success.
Disclaimer -
The information provided in this podcast is for educational purposes only and should not be considered as financial or professional advice. The views and opinions expressed are those of the host and guests and do not necessarily reflect the views of any organizations mentioned. Listeners are encouraged to conduct their own research and consult with qualified professionals before making any business decisions. This podcast may contain forward-looking statements that are subject to risks and uncertainties. Actual results may differ materially from those projected.