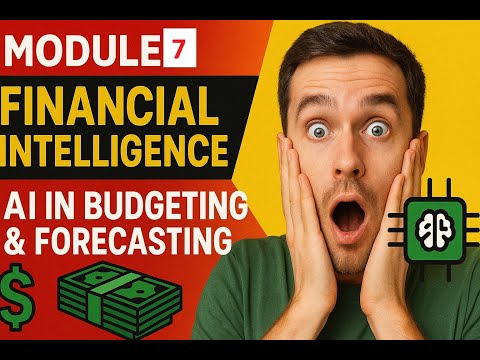
Module 7 Financial Intelligence: AI in Budgeting & Forecasting
AI-Powered Business & Management Mastery
#FinancialIntelligence, #AIForBusiness, #SmartBudgeting, #InvestmentAI,#BusinessForecasting, #FutureOfFinance, #DigitalTransformation, #AIInAccounting, #SuccessDriven, #GlobalBusinessInsights
We're diving much deeper, exploring the intricacies, the challenges, and the exciting future of AI in financial intelligence.
Imagine a multinational corporation headquartered in London, grappling with intricate global supply chains, fluctuating currency exchange rates, and the constant pressure to optimize financial performance across diverse markets. Now, envision a suite of intelligent AI systems that not only predict market shifts with remarkable accuracy but also proactively identify potential risks, optimize resource allocation in real-time, and provide strategic recommendations that drive sustainable growth. This isn't a distant vision; it's the evolving reality powered by AI in financial intelligence.
Let's delve into more detailed accounts of AI's transformative impact:
The E-commerce Platform's Journey to Proactive Financial Management
Consider a rapidly expanding e-commerce platform based in Jakarta, Indonesia. Before adopting AI, their finance team struggled with massive transaction volumes, leading to delays in financial reporting and reactive decision-making. Identifying this bottleneck, they implemented an AI-powered financial management system. This system, leveraging machine learning algorithms, automatically categorized thousands of daily transactions with high accuracy, reconciled payment gateways in near real-time, and generated dynamic financial reports. The implementation wasn't without its hurdles; initially, data inconsistencies required careful cleaning and mapping. However, once the AI models were trained, the impact was significant. Month-end closing times were reduced by over 60%, allowing the finance team to shift their focus from data entry to strategic analysis. Furthermore, the AI system began identifying subtle patterns in customer behavior and purchase trends, enabling more accurate sales forecasting and optimized inventory management, ultimately leading to a 15% increase in profitability within the first year.
The Healthcare Provider That Enhanced Financial Sustainability
In the United States, a large healthcare provider with multiple facilities faced challenges in managing complex billing processes, predicting patient volume, and optimizing resource allocation. They integrated AI-driven analytics tools that could process vast amounts of patient data, insurance information, and operational costs. Initially, there were concerns about data privacy and security, requiring robust anonymization and compliance protocols. However, the AI system proved invaluable in identifying billing errors, predicting peak patient demand, and optimizing staffing levels across different departments. This proactive approach not only improved revenue cycle management but also enhanced patient care by ensuring adequate staffing during critical periods, contributing to long-term financial sustainability.
To truly understand the power of AI in financial intelligence, it's crucial to grasp some of the underlying techniques:
Machine Learning Algorithms: At the heart of many AI financial applications are machine learning algorithms.
Regression Analysis: Used for forecasting financial variables like sales revenue, stock prices, or interest rates based on historical data and influencing factors. For example, a regression model can analyze past marketing spend and sales figures to predict the impact of future campaigns.
Classification Algorithms: Employed for tasks like fraud detection classifying transactions as fraudulent or legitimate or credit risk assessment categorizing borrowers into different risk levels.
Time Series Analysis: Specifically designed for analyzing data points collected over time, crucial for forecasting financial trends, identifying seasonality, and predicting future performance based on historical patterns.
Neural Networks and Deep Learning: More complex algorithms capable of identifying intricate patterns in large datasets, often used for sophisticated tasks like high-frequency trading analysis or predicting subtle market movements.
Natural Language Processing NLP: NLP enables AI systems to understand and process human language. In finance, this is invaluable for:
Sentiment Analysis: Analyzing news articles, social media posts, and earnings call transcripts to gauge market sentiment towards specific companies or industries, as we saw in our earlier anecdote about the investment firm.
Information Extraction: Automatically extracting key financial data points from unstructured documents like financial reports or contracts, saving significant time and reducing manual errors.
The benefits of AI in financial intelligence extend across various business sizes and sectors:
Small and Medium-Sized Businesses SMBs: AI-powered accounting and budgeting tools, often cloud-based and affordable, can automate routine tasks, provide clear financial visibility, and offer insights that were previously only accessible to larger enterprises. For a small restaurant chain, AI could forecast ingredient costs and optimize purchasing based on predicted demand.
Manufacturing: AI can optimize inventory levels based on demand forecasts, predict equipment maintenance needs to avoid costly downtime, and analyze production costs to identify areas for efficiency improvements.
Healthcare: Beyond billing and patient volume, AI can analyze financial data related to drug costs, treatment outcomes, and operational efficiency to optimize resource allocation and improve financial performance.
Non-Profit Organizations: AI can assist in forecasting donation trends, managing grant budgets effectively, and identifying potential donors based on historical data and engagement patterns.
While the potential of AI in financial intelligence is immense, it's crucial to acknowledge the challenges and considerations:
Data Quality: The accuracy and reliability of AI models heavily depend on the quality of the data they are trained on. Inconsistent, incomplete, or biased data can lead to inaccurate predictions and flawed insights.
Integration Complexity: Integrating new AI systems with existing legacy financial infrastructure can be complex and require significant technical expertise. Ensuring seamless data flow and system compatibility is crucial.
The Need for Skilled Personnel: While AI automates many tasks, it also creates a need for professionals who can understand, interpret, and manage these AI systems. Financial analysts and data scientists with AI skills are increasingly in demand.
Ethical Considerations and Bias: AI models can inadvertently perpetuate biases present in the training data, leading to unfair or discriminatory financial outcomes. Ensuring fairness, transparency, and accountability in AI algorithms is paramount.
The Importance of Human Oversight: Despite the power of AI, human judgment and expertise remain essential. AI-driven insights should be carefully reviewed and validated by financial professionals who understand the broader business context.
The field of AI in financial intelligence is rapidly evolving, with several exciting trends on the horizon:
Explainable AI XAI: As AI models become more complex, the need for transparency and interpretability is growing. XAI aims to develop AI systems that can explain their reasoning and decision-making processes, making them more trustworthy and easier to audit.
Predictive Analytics for Risk Management: AI is increasingly being used to predict and mitigate financial risks, such as credit default, market volatility, and even potential fraudulent activities, with greater accuracy and speed.
Hyper-Personalized Financial Planning: AI could enable highly personalized financial planning services tailored to individual needs and goals, offering customized budgeting advice, investment strategies, and retirement planning scenarios.
The Convergence of AI with Other Technologies: We'll likely see greater integration of AI with other technologies like blockchain for secure and transparent financial transactions and the Internet of Things IoT for real-time data collection that informs financial forecasting.
"The next wave of AI in finance won't just be about automation; it will be about augmentation. AI will empower financial professionals to perform higher-level strategic thinking by handling the heavy lifting of data analysis and prediction. However, it's crucial to remember that AI is a tool, and like any tool, its effectiveness depends on the skill of the user and the quality of the input. Ethical considerations and robust governance frameworks will be essential to ensure responsible AI adoption in the financial sector."
Let's revisit the integration process with more granular detail:
Step 1: Comprehensive Financial Landscape Assessment: Go beyond identifying pain points. Conduct a thorough audit of all financial data sources, data formats, and existing workflows. Map data dependencies and identify potential data silos that could hinder AI implementation.
Step 2: Strategic AI Tool Selection: Don't just focus on features. Evaluate the vendor's expertise, security protocols, data privacy policies, and the availability of robust support and training. Consider pilot projects with different tools to assess their suitability for your specific needs.
Step 3: Robust Data Connection and Transformation: Implement secure and reliable data pipelines. Invest in data cleaning and transformation processes to ensure data quality and consistency before feeding it into AI models. This may involve data normalization, handling missing values, and resolving data inconsistencies.
Step 4: Granular Metric Definition and Alert Customization: Define not just key metrics but also the specific thresholds and conditions that should trigger alerts. Customize alert delivery mechanisms to ensure timely and relevant notifications to the appropriate stakeholders.
Step 5: Iterative Monitoring, Validation, and Model Retraining: Implement continuous monitoring of AI model performance. Establish processes for validating AI-driven insights and predictions against real-world outcomes. Plan for regular model retraining with new data to maintain accuracy and adapt to evolving business conditions.
It's natural to have questions and concerns about the increasing role of AI in finance:
Job Displacement: While AI will automate many routine tasks, it's more likely to augment human roles rather than completely replace them. Financial professionals will need to adapt their skills to focus on strategic analysis, interpretation of AI insights, and managing the AI systems themselves.
The "Black Box" Problem: Some advanced AI models, particularly deep learning networks, can be difficult to interpret. This is where the development of Explainable AI XAI is crucial, aiming to make AI decision-making more transparent and understandable.
Security Risks: Handling sensitive financial data with AI systems requires robust security measures to prevent data breaches and cyberattacks. Choosing reputable vendors with strong security protocols is essential.
The integration of AI into financial intelligence is not just a technological advancement; it's a fundamental shift in how businesses understand, manage, and leverage their financial data. By embracing AI, organizations can move from reactive reporting to proactive prediction, from manual processes to automated efficiency, and ultimately unlock deeper insights that drive sustainable growth and competitive advantage in an increasingly complex global landscape. The AI edge in financial intelligence is no longer a luxury—it's becoming the key to future business success.
Disclaimer:
This podcast is for educational purposes only. It does not constitute financial advice. Please consult with a certified financial advisor before making investment or budgeting decisions. Mentioned tools and platforms are cited for illustrative purposes only; we are not affiliated with or sponsored by any brands listed.